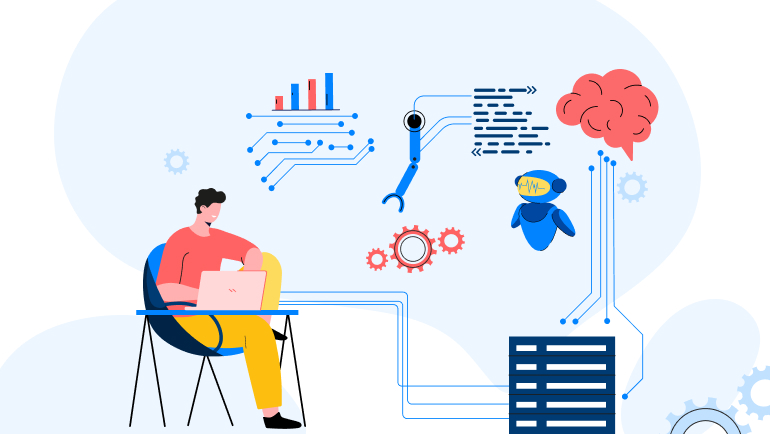
Your AI performance is as good as its data. AI tools depend fully on its data to identify patterns, predict results, and advise. Hence, the effectiveness of AI-powered results is heavily anchored to the quality of data we utilize.
In HR, we leverage AI to gauge employees’ sentiments, identify key talent fit for the role, and determine emerging skills to make decisions, deploy strategies, and future-proof the organization. Using a poor, unreliable dataset to train the AI model to help with these talent and business decisions could lead to misinterpretations and, in some cases, irrevocable consequences for the organization.
Quality data aids AI in this endeavour, creating a partnership where AI boosts HR's capacity to engage and understand employees and talent market. This relationship highlights the imperative for organizations to invest not just in AI-powered HR technology but also in ensuring HR data quality.
Why is Data Quality Paramount in AI-Driven HR Platforms?
The quality of HR data organizations collect, store, and use in training AI models reflect its data integrity. This, in turn, ensures that your HR data is fully reliable and applicable to derive insights that help make right business and talent decisions.
- Decision-Making Foundation: Quality data optimizes AI's decision-making for HR and managers, from recruitment to performance evaluations. However, poor data quality can lead to flawed analyses. For instance, inaccurate data on employee performance metrics might result in undeserved promotions or overlooked talents, affecting team morale and productivity.
- Employee Experience Understanding: Quality data reflects the diverse sentiments of today's evolving workforce. With it, AI provides contextualized insights for tailored, effective talent strategies. If HR misinterprets experience feedback due to poor data quality, they may implement initiatives that do not address actual concerns, leading to decreased job satisfaction and higher turnover rates.
- Bias Prevention: Quality data ensures AI-driven HR decisions are fair, unbiased, and objective. Preventing harmful biases from corrupted data is essential to promote a balanced workplace.
- Compliance Checks: Quality data ensures all HR-related regulatory and compliance checks are in place. However, inaccurate employee data can lead to violations of labour laws or reporting requirements, potentially resulting in fines and legal actions.
- Cost Efficiency: Quality data optimizes employee costs with all the checks and balances in place. For example, mistakes arising from low-quality rewards data can lead to salary discrepancies, like overcompensation or under compensation, creating financial inefficiencies in the organization.
Quality HR data ensures high data integrity, which leads to right decision-making and building employees’ trust in the organization and HR. But the consequences of not maintaining data quality while using AI are severe. Any errors due to bad data can hamper employees’ experience and erode their trust in HR, making them less likely to engage with or rely on HR initiatives and systems. Meanwhile, HR and managers will also be discouraged to use AI-powered tools leading to decreased productivity and a halt in the digitizing HR.
Common Reasons Behind the Deterioration of Data Quality
- Human Errors: Whether unintentional typos or malicious alterations, human mistakes can severely impact data quality. Regular training and vigilant monitoring can mitigate such risks.
- Transfer Errors: Data transfers, if not executed correctly, might corrupt or misdirect vital information. Secure and verified transfer protocols can prevent these issues.
- Cyber Threats: With increasing cyber-attacks, protecting data from threats like malware and ransomware is critical to maintaining data integrity.
- Security Issues: Unauthorized access to data does not only create security issues but also hamper the data quality, risking its integrity. Applying latest security configurations and patching any vulnerabilities are crucial to addressing such security issues.
- Hardware or Infrastructure Issues: Hardware issues such as physical failures can lead to data loss or corruption. Investing in reliable and secure hardware and infrastructure is essential to avoid such issues.
Principles for Ensuring Data Quality in AI
There are six key criteria to ensure quality data is being used in your AI engine. Each of these criteria can be achieved through specific methods and tools to maintain high level of data integrity.
Data Quality Criteria |
Description |
Methods and Tools to Ensure Quality |
Completeness |
Comprehensive datasets covering all required elements |
Regular data audits |
Consistency |
Data is consistent across all systems |
Data normalisation |
Uniqueness |
No redundant data in storage systems |
Using deduplication tools |
Timeliness |
Data reflects current situations and is timely |
Real-time data processing |
Validity |
Data is verified for correctness and structure |
Apply data validation rules |
Conformity |
Data aligns with organizational standards |
Adherence to data standards |
How Darwinbox Maintains Data Integrity in its AI-Powered HR Platform
- Data Validation: At Darwinbox, each piece of data is rigorously scrutinized. We compare all inputs against established standards and guidelines, ensuring only accurate information makes its way into our system.
- Data Cleaning and Pre-processing: Aware of the potential discrepancies in raw data, Darwinbox utilizes state-of-the-art data purification techniques. This process irons out any inconsistencies, anomalies, or inaccuracies, resulting in pristine datasets ready for in-depth analysis.
- Data Labelling: Given the significant role of AI and machine learning in HR analytics, data labelling is paramount. At Darwinbox, we take extra care in assigning appropriate labels to data, ensuring our analytical models operate with clarity and precision, thus yielding insightful results.
- Data Backup and Recovery: We prioritize data safety at Darwinbox. Frequent backups and robust recovery protocols ensure that even in unforeseen data loss events, the essence of our information remains intact.
- Feedback Mechanisms: Darwinbox values the insights and experiences of its users. Through established feedback channels, we keep refining our data practices. Additionally, we regularly consult with clients’ leadership team to ensure our data processes align with organizational needs and goals.
- Data Redundancy Checks: To preserve the uniqueness of our datasets, we employ advanced deduplication systems. This continuous scan keeps our databases free from repetitive records and guarantees the singularity and timeliness of each entry.
Conclusion
In a world where AI is becoming a vital tool for HR and business decisions, the quality of data really matters. Darwinbox understands this and has set up thorough processes to make sure our data is top-notch. From checking and cleaning data to listening to user feedback, we are committed to getting it right. As AI grows and becomes even more central to HR, we at Darwinbox will keep putting data quality first. It's about making smart and right decisions that benefit everyone in the workplace.
To understand our data quality checks and processes in more detail, schedule a call with our experts here.
Speak Your Mind